- On Target Date Investing
- T. Rowe Price’s Glide‑Path Design Framework
- An investment and behavioral solution.
- 2023-05-19 00:38
-
Kimberly DeDominicis, Portfolio Manager, Target Date Strategies
Andrew Jacobs Van Merlen, CFA, Portfolio Manager, Target Date Strategies
Wyatt Lee, CFA, Head of Target Date Strategies
Louisa Schafer, Ph.D., Senior Quantitative Investment Analyst
James A. Tzitzouris, Ph.D., Director of Research, Multi-Asset - Key Insights
-
- Behavioral preferences and objectives are as critical to the glide‑path design process as capital market assumptions and participant demographics.
- The robustness of our framework stems from realistic modeling of the economic environment, participant behavior, and plan sponsor and participant preferences.
- Our primary goal is to balance the trade‑off between limiting portfolio variability and supporting retirement consumption for all the participants in a plan.
Glide‑path design happens at the intersection of financial and behavioral economics. This is because retirement outcomes depend not only on investment returns, but also on the saving and spending behaviors and attitudes of investors. Therefore, an effective approach to life‑cycle investing must rely on a deep understanding of markets and investor behavior, including how both factors evolve and interact over extended time horizons and a wide range of market and economic cycles.
In order to properly assess the impact and interaction of these elements, T. Rowe Price employs a structural model for glide‑path design and evaluation. The primary benefit of our model is the consistency of our approach.
"The primary benefit of our model is the consistency of our approach."
We recognize that while individuals may be skillful at using intuition and judgment to solve complex problems, they may not be as effective at applying these skills consistently or on a broad scale. By contrast, our structural model allows us to apply our insights consistently across the range of glide‑path problems that we seek to solve.
Our evaluation framework is based on a utility model that incorporates key variables of glide‑path design and provides a consistent mechanism for assessing different potential outcomes based on varying assumptions about plan sponsor and participant preferences and objectives.
Key Design Factors
The first step in understanding how we apply our framework is to understand the primary factors that can influence glide‑path design.
A Consistent Model for Assessing Potential Outcomes
(Fig. 1) Key factors influencing glide‑path design
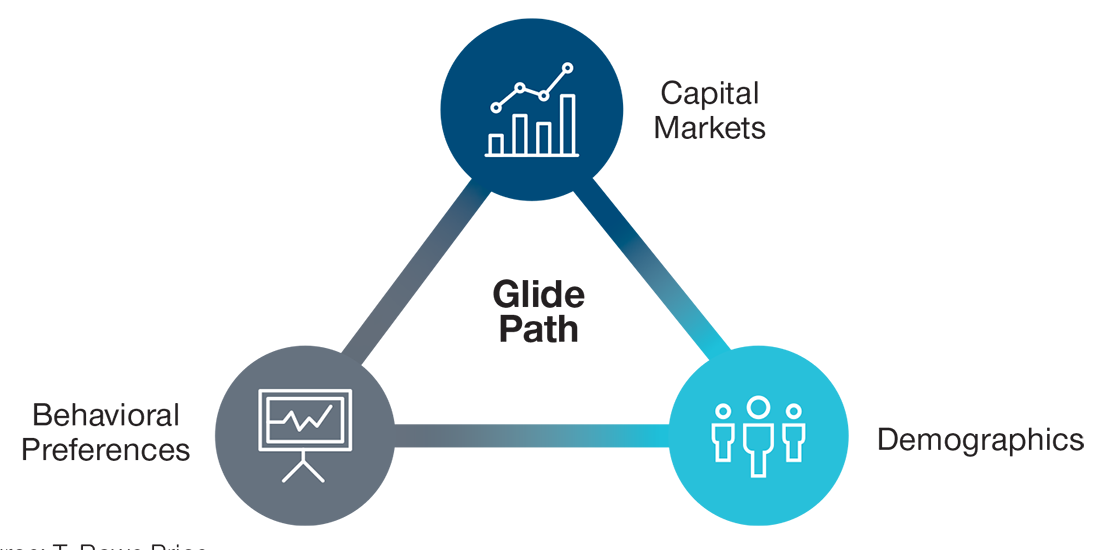
Source: T. Rowe Price.
There are three categories of relevant factors (Figure 1). Capital markets and demographics are assumptions about asset class returns and the funded status of the investors for whom the glide path is being designed. Behavioral preferences capture biases and goals pertaining to risk, avoiding wealth depletion, time horizon, and consumption.
- Capital markets: These are assumptions about expected future asset class returns, which are informed by economic variables such as growth, inflation, and interest rates.
- Demographics: Demographic assumptions are crucial to modeling projected investor cash flows, such as income, savings, and expenditures. Our model includes variables such as earnings (which are dependent on career progression and the state of the economy), income‑dependent savings rates, employer‑match formulas, and expected Social Security benefits, as well as mortality rates and behaviorally representative patterns of retirement spending.
- Behavioral preferences: Behavioral preferences determine how investors rank and compare investment decisions and outcomes. These preferences reflect investors’ attitudes toward uncertainty, wealth depletion, and the timing of consumption.
Modeling behavioral preferences allows us to intuitively capture investors’ unique tastes, construct objective criteria, and apply a consistent investment evaluation process across a variety of retirement goals and expectations. Our framework focuses on two categories of behavioral preferences: innate preferences and objective preferences.
Innate Preferences
Innate preferences are those ingrained for a chosen individual, which cannot be easily changed and are not objectives to be set. In our model, we assume two innate preferences: the degree of risk aversion and the degree of wealth depletion aversion.
- Risk aversion describes an individual’s willingness to trade a level of expected consumption for its greater certainty. More risk‑averse investors typically are willing to forgo some potential income in exchange for a higher likelihood of attaining a more modest income goal.
- Wealth depletion aversion captures an individual’s willingness to forgo current consumption in order to try to maintain their current wealth. This inclination to try to preserve wealth is not entirely driven by the need to fund future consumption. Rather, greater wealth is its own distinct source of satisfaction in our model.
Higher Risk Aversion Tends to Cause the Desired Glide Path to Shift Downward
(Fig. 2) Impact of risk aversion
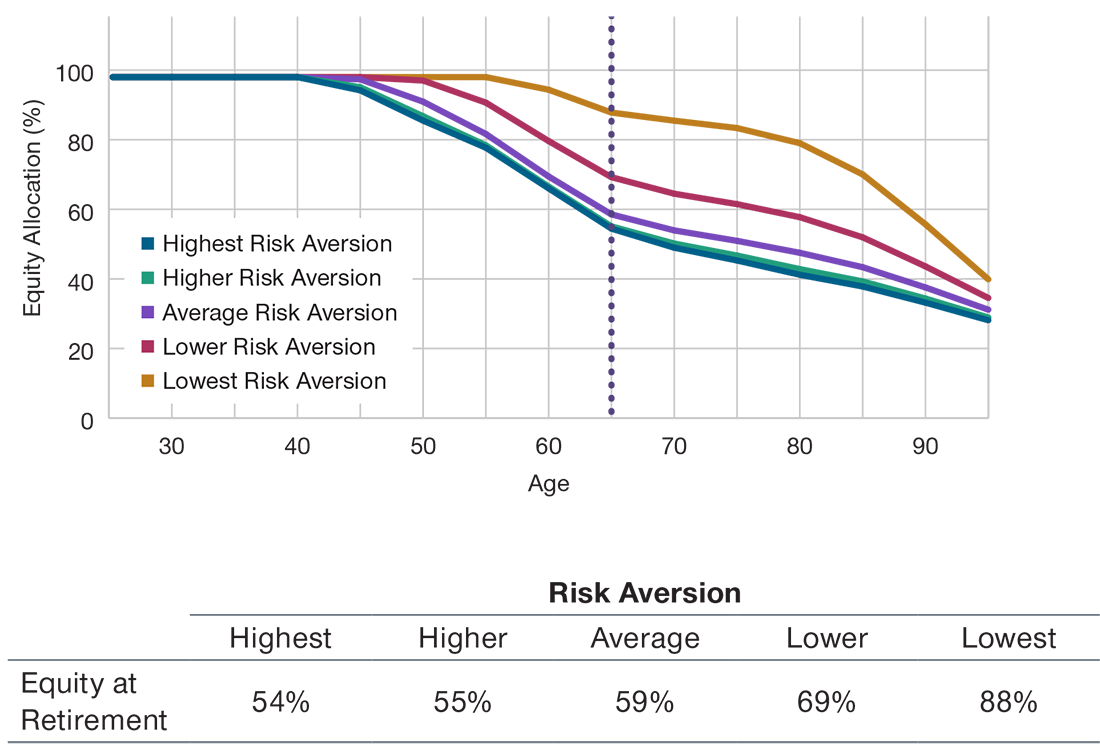
Source: T. Rowe Price.
For illustrative purposes only. Not representative of an actual investment or T. Rowe Price product. This is not intended to be investment advice or a recommendation to take any particular investment action.
This analysis contains information derived from a Monte Carlo Simulation. See Additional Disclosures for more information.
Academic research suggests that investors’ risk aversion is inherent and does not change over their lifetimes. Given the challenges in correctly eliciting risk aversion preferences1 and our goal of representing diverse participant populations,2 we model risk aversion not as an oversimplifying single parameter but as a distribution of potential values. This allows us to consider a range of potential investor preferences in our decision‑making process.
Aversion to wealth depletion is the other key innate preference parameter in our framework. An investor with no aversion to wealth depletion and a known retirement horizon typically would be expected to consume all their wealth over their lifetime. However, empirical research has shown that many retirees decumulate assets slowly, if at all.3 Potential explanations for such behavior are worries about longevity risk, a desire to self‑insure against possible medical and long‑term care expenses, a bequest motive, or a general difficulty in transitioning to “decumulation mode.”
The depletion aversion parameter explicitly characterizes this observed penchant for liquidity. Furthermore, it seeks to prevent the hypothetical participants in our model from “blindly running into a brick wall” and depleting all their wealth, which can happen under naive spending rules.
In our model, wealth depletion aversion is tied to the salaries and spending habits of plan participants:
- Investors with higher salaries tend to have a greater portion of their budgets dedicated to discretionary expenditures. Consequently, their consumption replacement needs in retirement may be lower than for lower‑income participants.
- A higher salary also suggests greater ability to save and a reduced focus on immediate consumption.
Together, these factors point to high earners having a stronger preference for avoiding balance depletion.
Risk aversion significantly influences the level and shape of the designed glide path. Unsurprisingly, higher risk aversion tends to cause the glide path to shift downward—i.e., the desired equity allocation tends to decline (Figure 2).
Greater depletion aversion tends to lead to less aggressive decumulation of wealth through retirement. Because it lowers postretirement spending levels and, thus, raises the funded status, a greater wealth depletion aversion also can result in a relatively lower equity glide path, all other things being equal.
Objective Preferences
Objective preferences are defined by plan sponsors on behalf of their participants and are determined by the investment goal over a certain time horizon.
The goal parameter captures the trade‑off between the two main objectives of a plan sponsor: limiting portfolio balance variability for participants (especially in the “red zone” around retirement) versus seeking to provide a higher average level of consumption in retirement.
"The investment goal parameter and the distribution of planning horizons in our model both can be calibrated to the specific preferences of the plan sponsor."
These objectives are contravening in that glide paths with higher levels of equity assets should tend to improve consumption due to higher expected returns over the long run, but also may detract from the portfolio balance variability objective due to higher expected volatility along the way. While both objectives are undoubtedly important, different sponsors may weigh their relative importance differently. Some may weigh the objectives neutrally, others may lean toward emphasizing the consumption objective, while still others may favor emphasizing the portfolio balance variability objective. As the priority moves toward striving to maintain a relatively stable balance, the desired glide path tends to shift downward (Figure 3).
It is essential to assess the relative importance that the plan sponsor places on these two objectives because the answer affects the glide‑path design significantly.
Seeking to Limit Balance Variability and Supporting Higher Consumption Are Potentially Conflicting Goals
(Fig. 3) Impact of relative goal importance
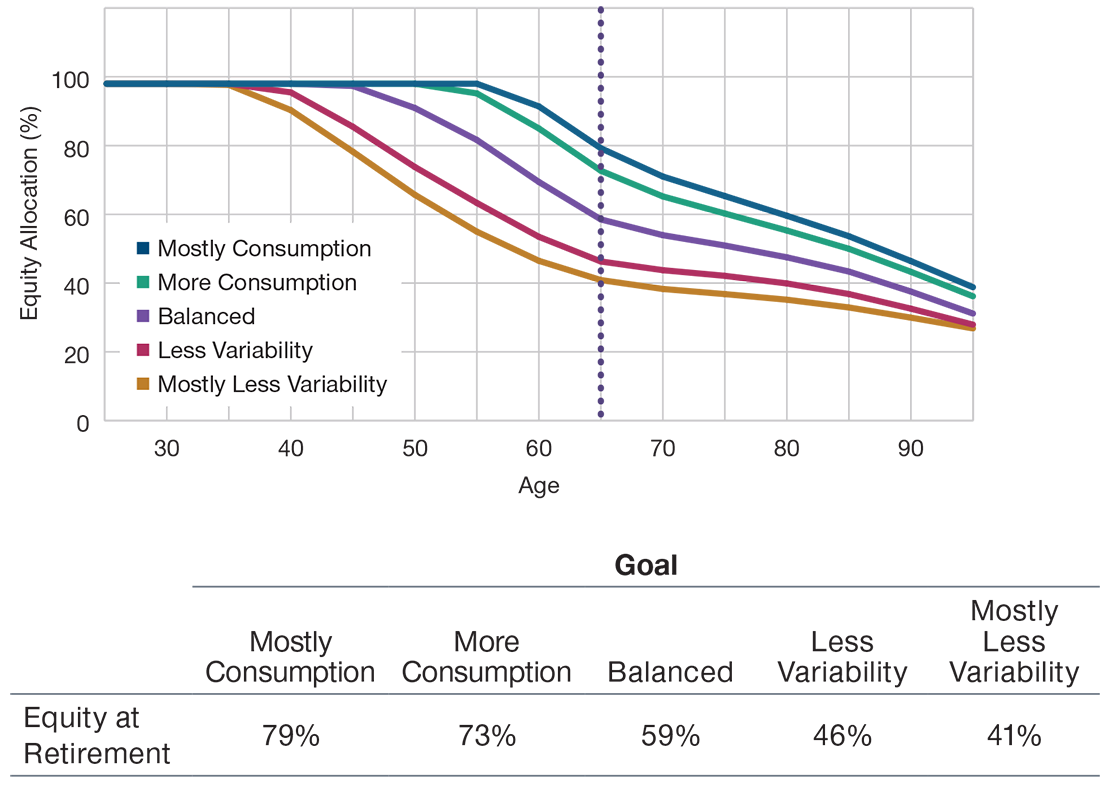
Source: T. Rowe Price.
For illustrative purposes only. Not representative of an actual investment or T. Rowe Price product. This is not intended to be investment advice or a recommendation to take any particular investment action.
This analysis contains information derived from a Monte Carlo Simulation. See Additional Disclosures for more information.
Another variable plan sponsors need to keep in mind is the planning horizon of their participants, which determines how individuals wish to distribute spending throughout their retirement years. More patient investors are willing to postpone consumption later into retirement. This preference is captured in our utility model by a participant‑specific discount factor applied to a satisfaction score. Computing duration using this time‑preference discount factor gives us a specific planning horizon for each hypothetical individual in a modeled scenario.
In our view, it is appropriate to consider glide‑path design and retirement income generation as planning problems, not actuarial pricing problems. In other words, target date investors tend to be more comfortable planning for their expenses to be covered up to a certain age. An actuarial approach may make sense for a defined benefit plan, where mortality risk and longevity risks are pooled. However, in a defined contribution (DC) plan, longevity risk is fully borne by the individual saver.
In line with this view, our model assumes that, at minimum, participants in retirement survive until their specific planning horizon. Beyond that period, we apply conditional mortality weights to adjust for the lower likelihood of being alive at older ages. For example, if an individual has a conditional retirement planning horizon of 23 years, in that scenario we would use mortality probabilities that are conditional on the participant living to age 88 (23 years after age 65). The model is calibrated such that the average conditional age is 85. Thus, the aggregate discount factor applied to the utility scores in our model can be thought of as the combination of two different discounts:
- The first component exclusively captures the individual investor’s patience.
- The second component is a mortality discount conditioned on an investor surviving up to their planning horizon, which is determined by the first component.
As far as we can tell, this combined approach is unique to our process. In our view, it should offer plan sponsors and participants an additional level of comfort when it comes to seeking to address longevity risk.
Planning horizon preferences influence not only how we compare investment outcomes, but also how we simulate investors’ spending behavior. Other things being equal, a longer planning horizon is associated with lower consumption earlier in retirement as individuals seek to let their balance grow before drawing heavily from them. As the planning horizon lengthens, however, the desired glide path tends to shift upward (Figure 4).
In contrast, shorter planning horizons encourage participants to front‑load their spending. In the extreme case, an individual might choose to consume their portfolio balance in one period—i.e., by withdrawing and spending their plan savings in a single lump sum.
Importantly, the investment goal parameter and the distribution of planning horizons in our model both can be calibrated to the specific preferences of the plan sponsor. By explicitly incorporating these parameters into our model, we seek to help plan sponsors express their target date objectives in a consistent, robust, and intuitive way.
Demographics Matter
In our model, the willingness of investors to allocate wealth to risky assets, such as equities, during the accumulation stage is most dependent on the ratio of their current portfolio balances to their annual contributions (including both voluntary savings and any employer matching contributions). As this ratio increases, the indicated glide path tends to shift downward.
Keeping this ratio in mind is also useful when considering how an isolated demographic characteristic change can influence glide‑path design. For example, consistently higher savings rates throughout the accumulation stage typically result in larger portfolio balances—and, thus, a higher ratio of balances to contributions. This tends to lower the desired glide path (Figure 5).
A Longer Planning Horizon Tends to Raise the Desired Glide Path
(Fig. 4) Impact of average planning horizon (APH)
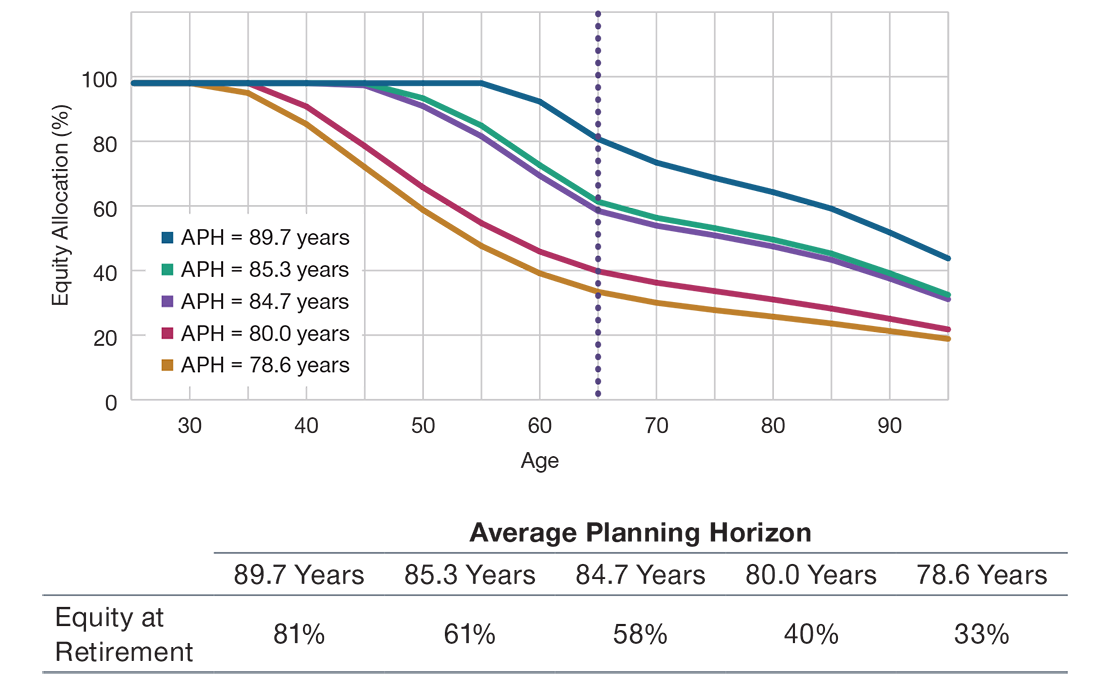
Source: T. Rowe Price.
For illustrative purposes only. Not representative of an actual investment or T. Rowe Price product.
This analysis contains information derived from a Monte Carlo Simulation. This is not intended to be investment advice or a recommendation to take any particular investment action. See Additional Disclosures for more information.
Higher Savings Rates Tend to Lower the Desired Glide Path
(Fig. 5) Impact of demographics
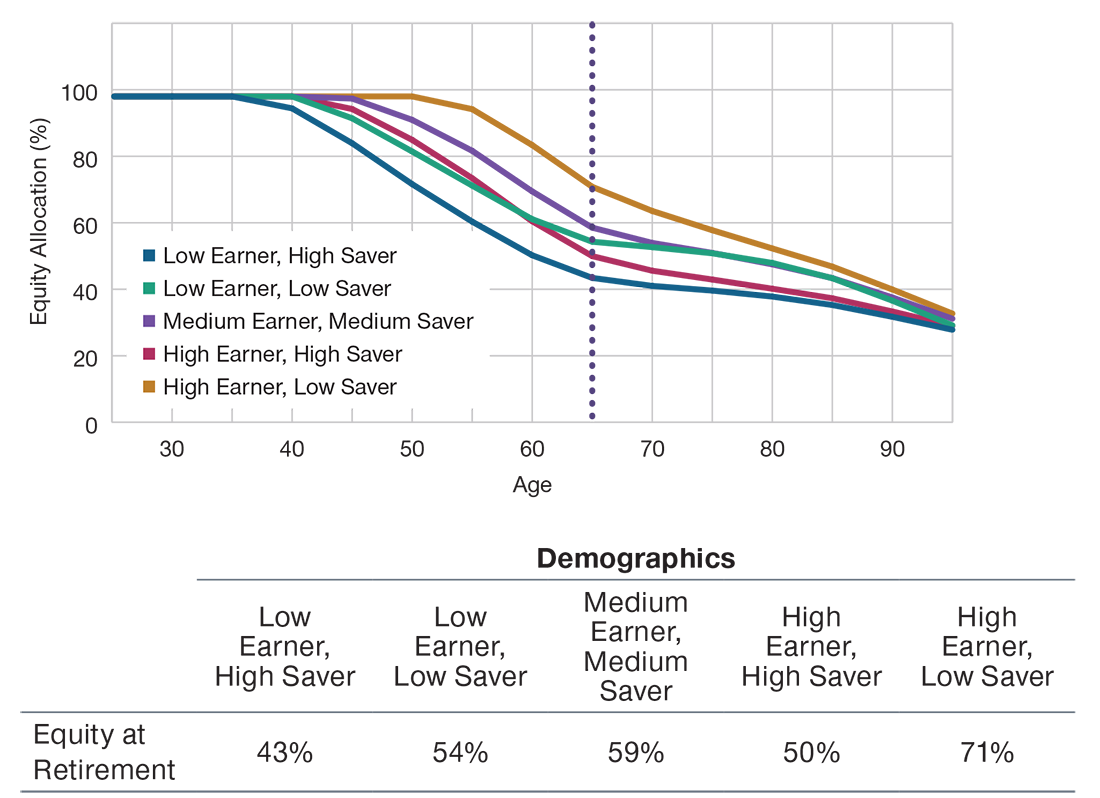
Source: T. Rowe Price.
For illustrative purposes only. Not representative of an actual investment or T. Rowe Price product.
This analysis contains information derived from a Monte Carlo Simulation. This is not intended to be investment advice or a recommendation to take any particular investment action. See Additional Disclosures for more information.
During the accumulation stage, we often use funded status—the ratio of wealth to salary—to track investors’ progress toward desired retirement outcomes. This metric has a strong positive correlation with the ratio of investors’ current balances to their annual contributions. As a result, a higher funded status (perhaps reflecting better investment performance and/or greater past contributions) implies a lower glide path in our model.
Our Design Framework
Having described the factors, we turn now to how those factors are incorporated into our design framework to produce a glide path. Our framework incorporates the key factors discussed above through a structural model consisting of three interconnected components (Figure 6).
- Economic scenario model (ESM): The ESM models the economy and capital markets, including indicators such as gross domestic product growth, price inflation, and real and nominal interest rates, as well as broad asset class returns.
- Behavioral scenario model (BSM): The BSM models the demographic characteristics that influence investor cash flow behavior. Since many of these behavioral elements also are influenced by what happens in the economy and the capital markets, the BSM draws upon the ESM.
- Utility model: The utility model incorporates a set of customizable behavioral preference parameters as inputs. This provides a scoring mechanism for the designed glide paths, allowing us to measure the satisfaction of plan participants and the sponsor in a consistent way.
An Emphasis on Robustness
An important feature of our design framework is our focus on making the process as robust and realistic as possible. Robustness is integrated into each component of our structural model.
"Robustness is integrated into each component of our structural model."
A glide path is a single predetermined asset allocation policy that is applied to a diverse set of plan participants across a wide breadth of market conditions. Thus, it is important for the glide‑path design process to consider heterogeneity in plan demographic characteristics and behavioral preferences.
Robustness Is Integrated Into Each Component of Our Structural Model
(Fig. 6) The big‑picture view
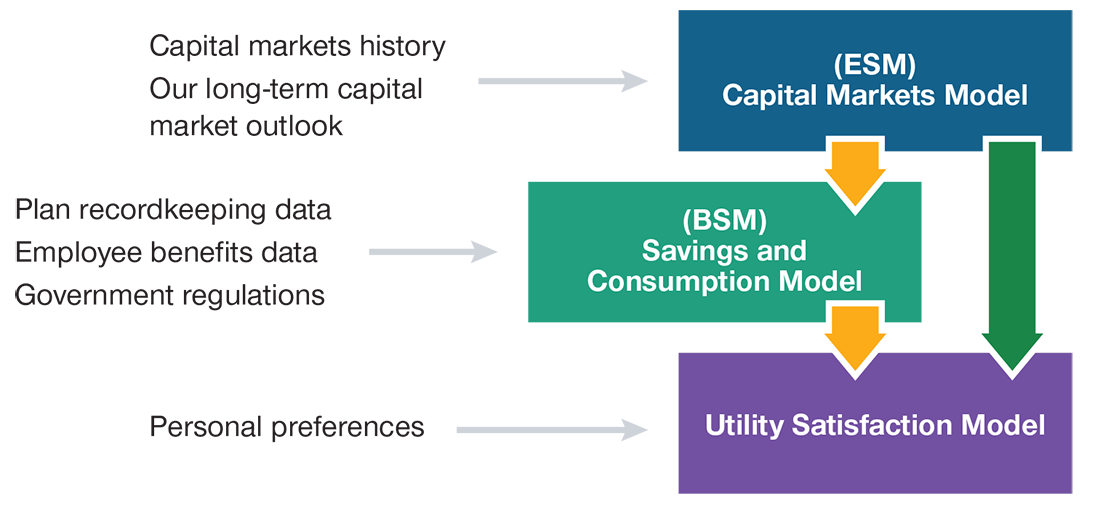
Source: T. Rowe Price. For illustrative purposes only.
Designing an asset allocation policy for the median or average participant is not the same as designing a policy for an entire plan. The latter requires proportionately representing plan subpopulations and carefully considering how an allocation policy could affect participants at opposite ends of the demographic and preference spectrums.
Our goal is not to design the perfect glide path for a specific individual (i.e., the median or average participant), but to try to provide the most appropriate and robust glide path for an entire plan population. We seek to achieve this by modeling demographic features and behavioral preferences not as oversimplifying single point estimates but as probability distributions of potential values. This should allow us to accurately characterize plan populations and model the nuanced trade‑offs between utilities of all participants within a plan.
We describe and discuss the potential benefits of a distributions‑based approach in a separate analysis, “Beyond Averages: A More Robust Approach to Glide‑Path Design.”4
Designing Glide Paths
Defining the investment objective is the first step in applying our structural model to glide‑path design. The objective preferences—the primary investment focus and the desired planning horizon—are determined by the plan sponsor. For example, the inputs for our Retirement Glide Path have been set to reflect that glide path’s primary objective: supporting lifetime income, with a secondary concentration on limiting balance variability around retirement. Both levers are a part of our utility model and can be calibrated using intuitive and comprehensible metrics, such as weighted balance volatility.
Since risk aversion and depletion aversion are innate to individuals, we do not adjust the distributions of these inputs in our model. In other words, we assume the typical ranges of risk aversion and depletion aversion. This method allows for accurate representation of participants’ needs and spending behaviors.
Next, we calibrate the demographic profile. For our proprietary solutions, the BSM is seeded with demographic information from T. Rowe Price’s DC recordkeeping platform. For customized glide paths, we can use the demographics for individual plans.
Once our inputs are set, the economic and behavioral models generate thousands of different scenarios. We then solve for the glide path that maximizes utility given the demographic inputs and the plan sponsor’s objectives. Our utility model not only scores the consumption of plan participants in retirement (a common approach), but also, by taking balance depletion aversion into account, recognizes the value that individuals place on having an asset cushion in line with their liquidity preferences and the inclination to preserve assets that we have observed empirically.
By aggregating individual utility scores, we seek to minimize the degree to which the utility of any subpopulation might otherwise be unfairly sacrificed for the sake of increasing the utility of another subgroup. Given that most plan populations are quite heterogeneous, this feature results in robust designs. Furthermore, at this step of the simulation, the objective preferences set by the plan sponsor meaningfully influence glide‑path design as components of the utility maximization process. The resulting asset allocation manages the nuanced trade‑off between portfolio balance variability and expected retirement consumption for all the participants in the plan.
How plan participants intend to spend their wealth throughout retirement has a major impact on the appropriate asset allocation. Consequently, spending assumptions can have a substantial effect on the level and shape of the glide path. Therefore, we have dedicated substantial time and effort to developing a model for retirement spending that is intuitive and consistent with empirical evidence.
Our utility model incorporates behavioral preferences when describing how investors compare glide paths. However, our spending model seeks to ensure that these preferences also reflect how investors actually behave. For example, we would expect investors with shorter planning horizons not only to value the ability to support higher spending earlier in retirement, but also to consume more of their wealth in their early retirement years. Thus, our preference‑driven spending model seeks to ensure consistency between investor desires and their expected spending behavior. Other potential benefits of our spending model include:
- It is rooted in established economic and financial models and explicitly captures the trade‑off between current spending and maintaining portfolio longevity to fund consumption in future retirement years.
- When determining an optimal level of spending, our model assumes that individuals consider a range of factors, including capital market expectations, life expectancy, balance history, Social Security benefits, and behavioral preferences.
- Our spending policy is a function of the current level of wealth, and its parameters are unique to each modeled participant and each retirement year. As a result, our process accounts for considerable heterogeneity in projected spending paths.
Since the current level of wealth is itself a function of market returns in past years, how much an investor spends can be expected to ebb and flow in accord with market performance. Unlike the “4%” rule of thumb, where the withdrawal amount is a fixed percentage of the preretirement balance, our spending policy is cognizant of past market events and expectations for future returns and, thus, generates realistic spending paths.
The final step of our process incorporates the insights and judgments of our portfolio management team. The insights that drive our glide‑path design process are rooted in fundamental and behavioral economics, as well as our institutional intuition, which has been informed by decades of experience managing life‑cycle strategies and acting as a provider and recordkeeper to retirement plans. The value of our systematic investment process lies in the way it permits the consistent application of these human insights in a scalable and repeatable way.
"...our depth and experience as an investment manager and a recordkeeper provide critical balance to our process."
While our models are effective at applying the themes and insights of our team across a population of investors, our depth and experience as an investment manager and a recordkeeper provide critical balance to our process. Our goal is to ensure that our model captures the benefits of our insights in the manner intended and in a way that reflects the needs of our clients. This feedback loop is essential to building and maintaining a robust glide‑path construction process.
References
Abel, Andrew B. 1990. “Asset Prices under Habit Formation and Catching up with the Joneses.” American Economic Review, 80 (2): 38–42.
Anderson, Lisa, and Jennifer Mellor. 2009. “Are Risk Preferences Stable? Comparing an Experimental Measure with a Validated Survey‑Based Measure.” Journal of Risk and Uncertainty, 39 (2): 137‑160.
Baker, Malcolm, and Jeffrey Wurgler. 2007. “Investor Sentiment in the Stock Market.” Journal of Economic Perspectives, 21 (2): 129–51.
Banerjee, Sudipto. 2018. “Asset Decumulation or Asset Preservation? What Guides Retirement Spending?” EBRI Issue Brief No. 447.
De Nardi, Mariacristina, Eric French, and John Bailey Jones. 2016. “Savings After Retirement: A Survey.” Annual Review of Economics, 8: 177–204.
Halek, Martin, and Joseph G. Eisenhauer. 2001. “Demography of Risk Aversion.” The Journal of Risk and Insurance, 68 (1): 1–24.
Holt, Charles A., and Susan K. Laury. 2002. “Risk Aversion and Incentive Effects.” American Economic Review, 92 (5): 1644–1655.
Kahneman, Daniel, and Amos Tversky. 1979. “Prospect Theory: An Analysis of Decision under Risk.” Econometrica, 47 (2): 263–291.
Zachary Rayfield and Kathryn Farrell. 2022. “Beyond Averages: A More Robust Approach to Glide‑Path Design.” T. Rowe Price Insights.
Noussair, Charles, N., Stefan T. Trautmann, and Gijs van de Kuilen. 2011. “Higher Order Risk Attitudes, Demographics, and Financial Decisions.” CentER Working Paper Series No. 2011–055. On the Web at http://ssrn.com/abstract=1843094.
-
FOR INVESTMENT PROFESSIONALS ONLY. NOT FOR FURTHER DISTRIBUTION.
1 Anderson and Mellor 2009 found that risk preferences are not stable across elicitation methods. Factors such as comprehension and effort affected risk preference stability. There is mixed evidence on whether risk aversion differs between hypothetical and paid‑out lotteries (Holt and Laury 2002; Noussair, et al., 2014). It is important to distinguish willingness to take investment risk from inherent risk preferences. Investment risk aversion can be influenced by habit formation (Abel 1990), loss aversion (Kahneman and Tversky 1979), and investor sentiment (Baker and Wurgler 2007). See the references section at the end of this paper for full citations.
2 Halek and Esienhauer 2001 found that age, gender, and race meaningfully affect individual risk aversion.
3 De Nardi, et al., 2016 and Banerjee 2018.
4 Zachary Rayfield and Kathryn Farrell. 2022. “Beyond Averages: A More Robust Approach to Glide‑Path Design.” T. Rowe Price Insights.
Additional Disclosure
Monte Carlo simulations model future uncertainty. In contrast to tools generating average outcomes, Monte Carlo analyses produce outcome ranges based on probability—thus incorporating future uncertainty. All exhibits are provided for illustrative purposes only.
Material Assumptions include:
- Underlying economic and behavioral inputs, including savings rates and cash flows, are generated from a structural model built up from factors relating to both financial markets and the broad economy as well as data calibrated based on T. Rowe Price’s recordkeeping platform’s participant population.
- The mortality weighting is sourced from the Society of Actuaries. Retirement age is assumed to be 65 years old.
Material Limitations include:
- The analysis relies on assumptions, combined with a return model that generates a wide range of possible return scenarios from these assumptions. Despite our best efforts, there is no certainty that the assumptions and the model will accurately predict asset class return ranges going forward. As a consequence, the results of the analysis should be viewed as approximations, and users should allow a margin for error and not place too much reliance on the apparent precision of the results.
Users should also keep in mind that seemingly small changes in input parameters, including the initial values for the underlying factors, may have a significant impact on results, and this (as well as mere passage of time) may lead to considerable variation in results for repeat users.
- Extreme market movements may occur more often than in the model.
- Market crises can cause asset classes to perform similarly, lowering the accuracy of our projected return assumptions, and diminishing the benefits of diversification (that is, of using many different asset classes) in ways not captured by the analysis. As a result, returns actually experienced by the investor may be more volatile than projected in our analysis.
- Asset class dynamics, including but not limited to risk, return and the duration of “bull” and “bear” markets, can differ than those in the modeled scenarios.
- The analysis does not use all asset classes. Other asset classes may be similar or superior to those used.
- Taxes, fees, and transaction costs are not taken into account.
- The analysis models asset classes, not investment products. As a result, the actual experience of an investor in a given investment product may differ from the range of projections generated by the simulation, even if the broad asset allocation of the investment product is similar to the one being modeled. Possible reasons for divergence include, but are not limited to, active management by the manager of the investment product. Active management for any particular investment product--the selection of a portfolio of individual securities that differs from the broad asset classes modeled in this analysis --can lead to the investment product having higher or lower returns than the range of projections in this analysis.
Modeling Assumptions:
- The primary asset classes used for this analysis are stocks and bonds. An effectively diversified portfolio theoretically involves all investable asset classes including stocks, bonds, real estate, foreign investments, commodities, precious metals, currencies, and others. Since it is unlikely that investors will own all of these assets, we selected the ones we believed to be the most appropriate for long-term investors.
- The analysis includes 10,000 scenarios. Withdrawals are made annually at the beginning of each year.
- IMPORTANT: The projections or other information generated by T. Rowe Price regarding the likelihood of various investment outcomes are hypothetical in nature, do not reflect actual investment results and are not guarantees of future results. The simulations are based on assumptions. There can be no assurance that the projected or simulated results will be achieved or sustained. The charts present only a range of possible outcomes. Actual results will vary with each use and over time, and such results may be better or worse than the simulated scenarios. Clients should be aware that the potential for loss (or gain) may be greater than demonstrated in the simulations.
- The results are not predictions, but they should be viewed as reasonable estimates.
-
Important Information
This material is provided for informational purposes only and is not intended to be investment advice or a recommendation to take any particular investment action.
The views contained herein are those of the authors as of April 30, 2023 and are subject to change without notice; these views may differ from those of other T. Rowe Price associates.
This information is not intended to reflect a current or past recommendation concerning investments, investment strategies, or account types, advice of any kind, or a solicitation of an offer to buy or sell any securities or investment services. The opinions and commentary provided do not take into account the investment objectives or financial situation of any particular investor or class of investor. Please consider your own circumstances before making an investment decision.
Information contained herein is based upon sources we consider to be reliable; we do not, however, guarantee its accuracy.
Past performance is not a reliable indicator of future performance. All investments are subject to market risk, including the possible loss of principal. All charts and tables are shown for illustrative purposes only.
T. Rowe Price Investment Services, Inc., distributor, and T. Rowe Price Associates, Inc., investment advisor. For Institutional Investors Only.
© 2023 T. Rowe Price. All rights reserved. T. ROWE PRICE, INVEST WITH CONFIDENCE, and the bighorn sheep design are, collectively and/or apart, trademarks or registered trademarks of T. Rowe Price Group, Inc.
202306-2905763
Get the full story.
We’re ready to share our latest thinking, but industry regulations require you to register or sign in to continue reading this article. Thank you for understanding.
Just one more step.
To continue, you are required to read and accept our Terms & Conditions. Thank you for understanding.
-
Important Information
This material is provided for informational purposes only and is not intended to be investment advice or a recommendation to take any particular investment action.
The views contained herein are those of the authors as of April 30, 2023 and are subject to change without notice; these views may differ from those of other T. Rowe Price associates.
This information is not intended to reflect a current or past recommendation concerning investments, investment strategies, or account types, advice of any kind, or a solicitation of an offer to buy or sell any securities or investment services. The opinions and commentary provided do not take into account the investment objectives or financial situation of any particular investor or class of investor. Please consider your own circumstances before making an investment decision.
Information contained herein is based upon sources we consider to be reliable; we do not, however, guarantee its accuracy.
Past performance is not a reliable indicator of future performance. All investments are subject to market risk, including the possible loss of principal. All charts and tables are shown for illustrative purposes only.
T. Rowe Price Investment Services, Inc., distributor, and T. Rowe Price Associates, Inc., investment advisor. For Institutional Investors Only.
© 2023 T. Rowe Price. All rights reserved. T. ROWE PRICE, INVEST WITH CONFIDENCE, and the bighorn sheep design are, collectively and/or apart, trademarks or registered trademarks of T. Rowe Price Group, Inc.
202306-2905763