June 2023 / GLOBAL EQUITIES
Generative AI Holds Promise and Peril for Investors
Looking beyond the hype to see opportunities in builders and toolmakers
Key Insights
- T. Rowe Price managers and analysts believe that generative artificial intelligence (AI) will reshape the investment landscape.
- Mega‑cap tech companies are likely to dominate the “foundation models” on which AI rests, but a range of other companies will reshape it—and be reshaped by it in turn.
- Chipmakers and other “tool” providers may be the primary beneficiaries of a burgeoning AI “arms race.”
People can’t stop talking about artificial intelligence (AI) lately—and many of them are in Silicon Valley’s executive suites. The biggest headline to emerge from our Investment Division’s most recent annual “Tech Tour” visit to Silicon Valley to meet with some of the technology industry’s leading executives was this: AI has reached a tipping point and is poised to transform the market landscape.
A New “Arms Race” in the Technology Sector
Time will tell, but November 30, 2022, might go down as one of the most significant days in the history of technology—perhaps even on par with Steve Jobs’ introduction of the iPhone on June 29, 2007. On that November day, a small San Francisco‑based company, OpenAI, launched ChatGPT, which soon took the world by storm.
What made ChatGPT especially compelling is its use of natural language processing and so‑called generative algorithms. These two branches of AI allow ChatGPT to synthesize information it finds on the web, put it in the context of its current “discussion” with the user, and then reorganize the information to provide an answer. As indicated by its name, generative AI creates new content, rather than just aiding human perception and understanding, as was the case with previous forms of AI.
By some metrics, it was the fastest rollout of any technology in history—within a week, ChatGPT had over 1 million users, OpenAI estimated, while many more were lined up for their chance to access the tool. News reports later suggested that it had gained 100 million users within two months—the fastest any application had reached that threshold. For context, it took Instagram 30 months to do the same.
According to Dom Rizzo, recently appointed as the sole portfolio manager of the Global Technology Equity Strategy, the leading mega‑cap technology companies were taken by surprise by the magnitude of the consumer response to ChatGPT. The result has been what he describes as an “arms race” to acquire new AI capabilities and refine existing ones.
The Key Innovations Behind Generative AI
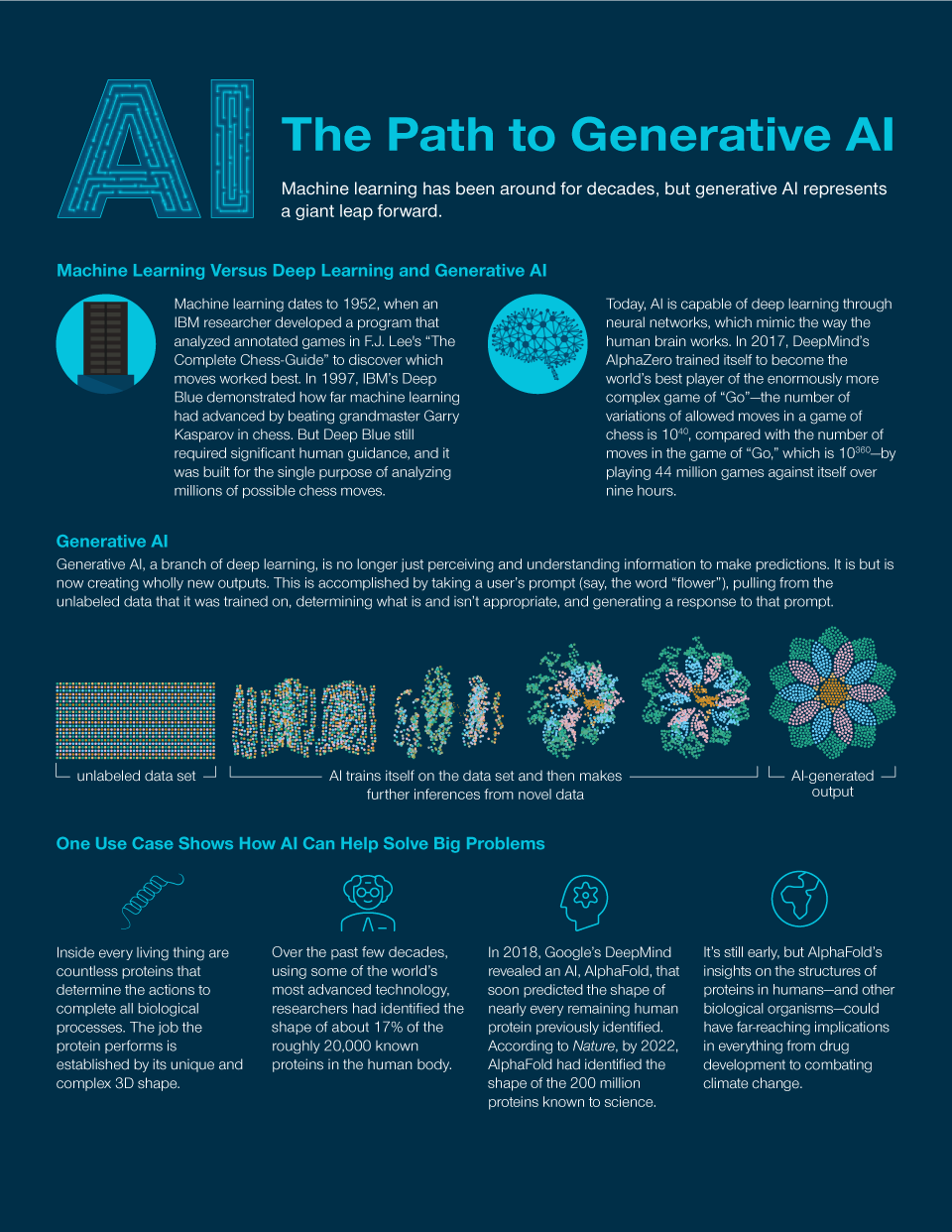
Our managers have long been following and investing in the basket of important and interrelated innovations that had to come together to build ChatGPT and other “foundation models.” These include cloud computing, new means of efficient communication between computing systems through so‑called application programming interfaces (APIs), and the accumulation of sheer computing power enabled by ever‑faster chips and processors.
Likewise, our managers and analysts suspect that a wide range of companies, both large and small, stand to benefit from the development of AI applications. And in our view, companies of nearly any kind—and their investors—need to pay attention.
In the popular culture, AI is often envisioned as a generalized system that has gathered all of the world’s information together and is capable of anything—whether benign or evil. But a more likely scenario is a small set of massive foundation models resting below thousands of specialized AI user interfaces—designed by perhaps nearly as many companies.
The AI Giants
To be sure, the technology giants that are household names have made some of the biggest leaps in developing AI’s fundamental models. Paul Greene, who manages the U.S. Large‑Cap Core Growth Equity Strategy, says the massive costs required to develop and run AI applications have been growing exponentially due to their complexity. (See Figure 1.)
The Exponential Growth (and Cost) of AI Complexity
(Fig. 1) More parameters* mean greater complexity—until recently*
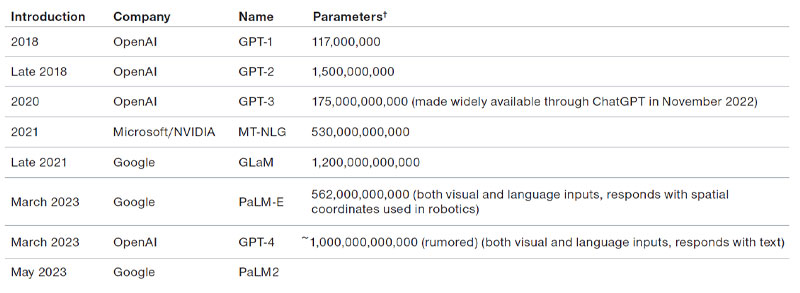
As of May 2023. For illustrative purposes only.
Sources: OpenAI, Google, NVIDIA, The Decoder, and TechTarget.
*Recently, some experts have questioned whether ever‑larger parameter counts would drive further advances in large language models and AI generally. Instead, Portfolio Manager Paul Greene notes that it seems likely that AI applications will grow more complex (and costly) as they are built to employ more models.
†A parameter is a variable contained within the model that is estimated by the analysis of historical data—typically, through machine learning and without human intervention. For example, the likelihood that the word “yellow” will be followed by the word “mustard” when “sandwich” is within a specified range might be one parameter in a natural language processing model.
Generative AI Isn’t Cheap
One reputable industry estimate is that it costs OpenAI a penny for each 30‑word answer to a question—a seemingly small sum that adds up quickly when the system has millions of users.1 Paul notes that a former Google AI specialist estimated the cost to train a homogenous, test‑only 530B parameter model to be USD 100 million. “There aren’t many companies that can write checks of this size,” Paul notes. “That includes OpenAI, which is being massively subsidized by its partner Microsoft.”
But the costs don’t end there. Paul also observes that while training models has been the biggest driver of expenses to date, it is widely expected that inferencing—or running the model to answer user queries—will be the costliest going forward. This sets AI apart from most other software, which can add users at negligible expense.
While rewarding scale, this is not necessarily all good news for the giants. “I worry about how the AI arms race will weigh on the balance sheets of even the mega‑caps,” Dom notes. “Alphabet, Microsoft, Meta, Amazon.com, and others are going to have to spend substantially to remain competitive in AI.”
Alphabet Appears to Remain at the Forefront—for Now
As another argument for scale, Paul points to the advantages of companies with massive, proprietary data sets, which they can deploy to train new models. Google parent Alphabet has special advantages in this regard, he believes. “Think of all their mapping data, app store data, and network data, as well as data collected through YouTube and all their many other products,” Paul argues. “These are all huge reservoirs of data that are extremely valuable on their own, and even more so when combined. Integration across products and logged‑in users creates cleaner data sets, and customers’ use of the products can itself help with automated labeling and human feedback.”
Paul believes that Google also has an important advantage in its talent pool. Several years ago, it was estimated that half of the world’s top AI experts worked for the company. While that figure has likely fallen since, Google probably maintains an outsized share of talent in this field. One of Google’s most important investments in AI came in its 2014 purchase of London‑based start‑up DeepMind, which came to the world’s attention in late 2015 when its “AlphaGo” program beat two of the world’s top players of the “Go” board game—a feat that was widely considered beyond the reach of computers.
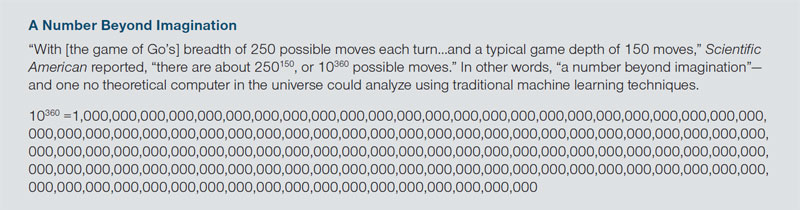
But Is Google’s Dominance Assured?
Shares in Alphabet suffered in the wake of ChatGPT’s release, seemingly in part because investors speculated that Google’s lucrative dominance of the internet search market would suffer from competition with ChatGPT and other “chatbots.”
Communications and Technology Equity Strategy Portfolio Manager Jim Stillwagon believes that Google search will evolve to incorporate more advanced AI capabilities over time. “We’re already seeing Google experiment with multimodal conversational formats alongside traditional text‑based blue links,” Jim notes. “With six products each serving over 2 billion users globally (Search, Gmail, Android, Chrome, YouTube, and Google Play), I believe Google will have significant distribution advantages in the race to scale and commercialize consumer‑facing AI services.”
That said, questions remain around the potential monetization and cost hurdles for search queries that require compute‑intensive large language models (LLMs) for AI‑generated responses. “Having spent the better part of three decades optimizing search results for link‑based lead generation,” Jim notes, “Google will likely have to adapt its advertising model to new search interfaces, while also balancing the additional cost burdens from LLM training and inference.”
Execution will matter, especially since the battle for search will be fought on Google’s home turf. Paul notes that this may prove to be a case of what’s known as the “innovator’s dilemma,” a term coined by Harvard’s Clayton Christensen for the tendency of companies that grow large in a new market to then lose it to—or be disrupted by—smaller firms. This is because large companies tend to focus on serving existing customers, while smaller and more nimble ones look to find new customers with new needs. Paul, Jim, and other managers and analysts at the firm are watching developments closely.
Leveraging Other Unique Data Sets
While it has largely kept its AI efforts under wraps, Amazon also stands to benefit from the dominance of its industry. The largest online retailer outside of China has its own unmatched reservoir of data on how consumers behave and think, through text (product reviews), verbal cues (responses to verbal assistant Alexa), movement (location data), and purchases of everything from books to medication.
Facebook’s decision to rename itself Meta Platforms and build out the “metaverse” reflects the company’s ambitions for the next generation of technology, including AI. Jim observes that Facebook has extensive experience in leveraging machine learning to understand its users and target appropriate advertising and content to the right people. As any parent can attest, TikTok has become a formidable competitor to Facebook in doing the same thing, perhaps even better.
Progress by Meta and Amazon in the development of generative IT remains unclear, although Dom notes that Meta has played an important role in building up the open‑source community for AI. “I certainly think that Meta has all the tools to be a leader in AI,” Dom notes, “and I believe Amazon’s huge cloud computing resources and internal semiconductor capabilities offer it a unique opportunity for building generative AI and bringing it to the masses.”
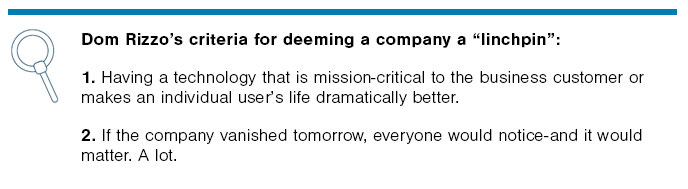
When Bing Meets Chatbot
The most immediate challenge to Google’s AI dominance is Microsoft—in large part because of its significant investment and partnership with OpenAI. Microsoft has already begun integrating chatbot into its Bing search engine and its Office software suite, and CEO Satya Nadella has announced ambitious plans to bring OpenAI deeper into the company’s software ecosystem.
As Paul notes, Microsoft is hardly a newcomer to AI. For example, Microsoft partnered with chipmaker NVIDIA to release the Megatron‑Turing Natural Language Generation model (MT‑NLG) in 2021, an important step in building generative AI. (See Figure 1.)
How much value generative AI and chatbots will add to Microsoft’s Bing or other voice‑interactive systems, such as Google Assistant, Amazon’s Alexa, or Apple’s Siri, remains to be seen. Alphabet stock plunged nearly 9% in early February after the rollout of its chatbot, “Bard,” incorrectly identified NASA’s new Webb telescope as the first telescope to take a picture of a planet outside of our solar system—a feat actually achieved in 2004. ChatGPT soon suffered its own embarrassment after trying to convince a New York Times writer to leave his wife for the program—a result of being trained on seductive text messages found on the web.
Investing in the Companies That Make AI Work
As the major companies pour money into AI, Dom sees a new AI bubble being formed—which could potentially even rival the dot‑com bubble of the late 1990s. This is one reason that Dom is focusing on the companies that he believes will be the “linchpins” in developing AI—those providing the indispensable tools for its further development. Somewhat like Levi Strauss and the other San Francisco merchants who supplied the miners during the California gold rush, the linchpins may actually benefit from overspending on AI.
At the top of Dom’s list are the companies central to progress making the advanced semiconductors necessary to assemble and run massive AI foundation models. At or near the top of this list, in Dom’s eyes, is chipmaker NVIDIA, whose graphics processing units (GPUs), originally designed for video games, have been key to the development of ChatGPT and generative AI. “We’re really at the beginning stages of an incredible revolution that’s based on accelerated computing power,” Dom says, “and I believe NVIDIA is leading the way.”
Dom explains that NVIDIA’s advantage came from its GPUs’ strength in parallel processing, which is central in building the complex neural networks that allow the rapid querying of vast data sets. “The best way to think about it is this analogy,” he says. “If you tasked a CPU [central processing unit] to find all the times Dickens wrote the word ‘the’ in ‘A Tale of Two Cities,’ the CPU would use serial processing and start on page 1 and then count: “It was the (1) best of times, it was the (2) worst of times....” The GPU, on the other hand, would quickly rip the book into 100 short sections and search those sections simultaneously for the word.
NVIDIA has also been adept at integrating itself into the broader AI software ecosystem. In 2007, the company released its Compute Unified Device Architecture (CUDA) application platform, which allows engineers to use common programming languages to design software operating on its parallel processing chips. More recently, the company extended CUDA to cooperate with open‑source software designed especially for AI applications.
Dom is also excited about the prospects for Advanced Micro Devices (AMD), a leader in both CPUs and GPUs. He points out that the company’s new chip, the MI300, is able to marry the benefits of the serial processing of a CPU and the parallel processing of the GPU.
Chips of All Kinds Will Feed the AI Revolution
But Dom isn’t only enthusiastic for the potential of AI chipmakers like NVIDIA and AMD. “The thing that excites me most is the general silicon intensity of AI,” he notes. “For example, an AI server can consume up to three times more DRAM [dynamic random access memory] than a traditional server and eight times more storage.”
Just as NVIDIA is behind the program labeled “ChatGPT,” so are other select companies behind computer chips labeled “NVIDIA”—or “Intel” or “AMD,” for that matter. A crucial one is Taiwan Semiconductor Manufacturing Corporation (TSMC), which has become a widely recognized leader in making ever‑smaller process nodes—a measure of how finely transistors can be etched onto silicon and, thus, the number of transistors can fit on a chip of a given size. Along with NVIDIA GPUs, Apple’s celebrated “Bionic” chips, which power the latest‑generation iPhones and Macbooks, are made by TSMC.
The Most Advanced Machines on Earth
But that’s only the beginning of a sophisticated production chain. In turn, TSMC’s current three‑nanometer process node would not be possible without the extreme ultraviolet (EUV) lithography machines developed by Netherlands‑based semiconductor equipment maker ASML Holding. It took 10 years and roughly EUR 10 billion for ASML to bring EUV to market, and Dom argues that its USD 200 million machines are probably the most advanced commercial technology in existence.
ASML’s EUV machines can operate at the three‑nanometer process node, which is only about nine times the diameter of a single gold atom. As AI demands ever‑more‑powerful chips, the limits of physics are being tested, and the end of the steady improvement in chip technology predicted by Moore’s law appears to be nearing.
For this reason, chip design is likely to become increasingly central to AI progress. Electronic design automation company Synopsys is a leader in chip design technology and appears to be centrally positioned to benefit from the growing demand. In an interesting feedback loop, Synopsys itself is using AI to help design chips that are faster and more power‑efficient.
Disruption Is Already Spreading Outward From Tech
Ironically, perhaps, the software industry itself seems to be among the first upended by AI. ChatGPT’s coding skills are formidable, and while it might not replace humans, Dom thinks it and other programs may enable many more 10x developers—industry jargon for the rare individuals who are 10 times as productive as their peers. Software engineers have long copied and shared batches of code using GitHub and other online resources, but tools like ChatGPT speed up writing code exponentially by doing much of that work themselves. As technology analyst Alan Tu notes, the result may be that the barriers to developing software are lowered, and what happens to the pace of innovation in the industry remains to be seen.
Health care is also seeing the early impact of AI advances. In late 2020, engineers at Google’s DeepMind revealed an AI, AlphaFold, that soon predicted the three‑dimensional structure of nearly every human protein based on its one‑dimensional chain of amino acids—chains of organic molecules that can run into the thousands.
Over the preceding half‑century, researchers had been able to identify the structure of only about 17% of the roughly 20,000 proteins found in the human body. As current U.S. large‑cap growth and former health sciences Portfolio Manager Taymour Tamaddon puts it, DeepMind essentially solved the protein folding problem.
T. Rowe Price’s health sciences team is closely watching how our new knowledge of protein structures and other AI advances will impact drug development and other medical fields. “Machine learning and AI have tremendous potential in the field of genomics,” according to biotechnology analyst Anne Daub, “as they can discern the patterns buried within massive and complicated genomics databases.” One example: Correlating genomics data and anonymous health records can lead to the discovery of pathogenic traits or, conversely, traits that are protective against disease. “Such data provide tremendous opportunities to design and develop drugs leveraging both aspects.”
Meanwhile, our analysts and managers across the firm are examining the possible implications of AI for a range of other sectors and industries—while keeping in mind the uncertainties created by the field’s unprecedented pace of change. “In tech, sometimes there’s just smoke, and sometimes there’s really fire,” as Dom puts it. “In AI, there’s really fire.”
Appendix
Securities mentioned in this material had the following weightings in the strategies' representative portfolios as of March 31, 2023. None of the securities mentioned were held in the representative portfolio of the Health Sciences Strategy.
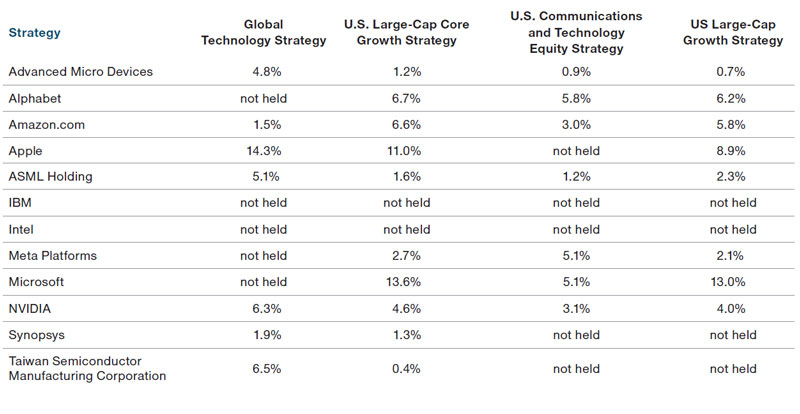
The specific securities identified and described do not represent all of the securities purchased or sold for advisory clients, and no assumptions should be made that investments in the securities identified and discussed were or will be profitable. Holdings are as of the date noted, may change at any time and are not recommendations to buy or sell any security.
The representative portfolio is an account we believe most closely reflects current portfolio management style for the strategy. Performance is not a consideration in the selection of the representative portfolio. The characteristics of the representative portfolio shown may differ from those of other accounts in the strategy. Information regarding the representative portfolio and the other accounts in the strategy is available upon request.
IMPORTANT INFORMATION
This material is being furnished for general informational and/or marketing purposes only. The material does not constitute or undertake to give advice of any nature, including fiduciary investment advice, nor is it intended to serve as the primary basis for an investment decision. Prospective investors are recommended to seek independent legal, financial and tax advice before making any investment decision. T. Rowe Price group of companies including T. Rowe Price Associates, Inc. and/or its affiliates receive revenue from T. Rowe Price investment products and services. Past performance is not a reliable indicator of future performance. The value of an investment and any income from it can go down as well as up. Investors may get back less than the amount invested.
The material does not constitute a distribution, an offer, an invitation, a personal or general recommendation or solicitation to sell or buy any securities in any jurisdiction or to conduct any particular investment activity. The material has not been reviewed by any regulatory authority in any jurisdiction.
Information and opinions presented have been obtained or derived from sources believed to be reliable and current; however, we cannot guarantee the sources' accuracy or completeness. There is no guarantee that any forecasts made will come to pass. The views contained herein are as of the date noted on the material and are subject to change without notice; these views may differ from those of other T. Rowe Price group companies and/or associates. Under no circumstances should the material, in whole or in part, be copied or redistributed without consent from T. Rowe Price.
The material is not intended for use by persons in jurisdictions which prohibit or restrict the distribution of the material and in certain countries the material is provided upon specific request.
It is not intended for distribution to retail investors in any jurisdiction.
June 2023 / MIDYEAR MARKET OUTLOOK